Understanding Artificial Intelligence And Machine Learning, Deep Learning article from Coding compiler – In this blog post, we’ll try to distinguish between artificial intelligence and machine learning and what’s their importance in data science.
Table of contents: AI, ML, DL
- The place of Artificial Intelligence and Machine Learning in the Data Science world
- What is Data Science?
- What is Artificial Intelligence – AI?
- Symbolic Artificial Intelligence
- What is Machine Learning – ML?
- What is Deep Learning – DL?
- Uses of Deep Learning in Industry
Before going into the details of Artificial Intelligence and Machine Learning, we’ll see how the two fields converge into the vast world of Data Science. Because this domain is rapidly changing, there is general disagreement about terms and definitions and some people may disagree with the use of other terms in the industry. So we’ll try to make some order.
Artificial Intelligence and Machine Learning in Data Science
Look at the below dolls. The first one is the largest doll, and inside it is another doll, slightly smaller so that you can enter the big doll. In the smaller doll is a little smaller doll, and so on.

With this analogy, we can describe the place of any subset in the world of Data Science. The largest doll represents the entire world Data Science, the second-largest represents the field of Artificial Intelligence, the next represents the field of machine learning, and last – Deep Learning.
What is Data Science?
Data Science investigates large amounts of data in order to bring business value and provide organizations with a competitive edge against competitors across all industries.
As an example, retail companies analyze consumers’ consumption habits to better target their marketing campaigns and bring the most effective deals to their customers.
In a reality where the amount of data is growing, it is important to have an effective Data Science strategy to help make informed business decisions. All of these areas have become more prominent, as there are more and more attempts to meet the growing demand for more effective ways to derive value from the diet.
The use of Artificial Intelligence to achieve these goals is a natural result of data evolution.
What is Artificial Intelligence – AI?
A little history:
Throughout the history of AI the definition has undergone several changes. In fact, Artificial Intelligence is a rather broad term and the idea started back in the 1950s. Machine Learning is a subset of AI and Deep Learning is a subset of ML.

The AI field began to be recognized and used as a research laboratory when a group of data scientists unveiled it at the Dartmouth Conferences in 1956. In the decades since, AI has been presented as the key to the glowing future of our civilization and encourages far-reaching technological development.
Over the past few years, AI has received a new life, especially since 2015.
The wide use of GPUs that enable parallel processing, fast, cheap and powerful, has greatly affected the AI input so intensely for processing data. It is also related to the incredible technological development in the field of data storage and of course the beginning of the era of Big Data with huge amounts of very diverse and unstructured data – images, text, transactions, data mapping, and more.
Today, Artificial Intelligence describes a machine/technology that can simulate and present human thinking behavior when making decisions or solving problems. We are talking about technologies that are capable of performing specific tasks, such as humans and even better. Examples of AI are, for example, categorizing images in Pinterest and facial recognition on Facebook. But how to do it?
The realization techniques include the use of Symbolic Artificial Intelligence, AI Planning, and of course Machine Learning.
Symbolic Artificial Intelligence
Symbolic artificial intelligence is one of the first approaches to AI based on the proposition that human understanding and learning can be achieved through the manipulation of symbols. This is the basis for symbolic systems, also called formal systems, that focus on three basic concepts that trace human thinking abilities:
- Symbol + plus is the result of connecting two vertical lines – it is immediately clear to the human mind what the essence of this symbol is – the connection.
- The thought is the pattern, for example, a sign plus means to put things together.
- The process of manipulation is actually an end-to-end line of thought – a product of combining symbols and patterns, such as we use the plus sign in a mathematical equation for one and two equals three.

What is Machine Learning – ML?
Now that we have an idea about Artificial Intelligence – building a system that can simulate human behavior, what about Machine Learning and how it converges within AI? The initial difference between the two is in the word “learning” since it is a learning system.
Machine Learning is actually a practice of using algorithms for analyzing data, running a learning process, and then providing definition and prediction for anything that is asked for. The system learns from large amounts of data to be able to perform tasks.
The algorithms can identify unique features within the data and then run a model that uses these features to predict new data. Machine Learning implementations are very effective in text analysis, speech analysis, image processing, and more.
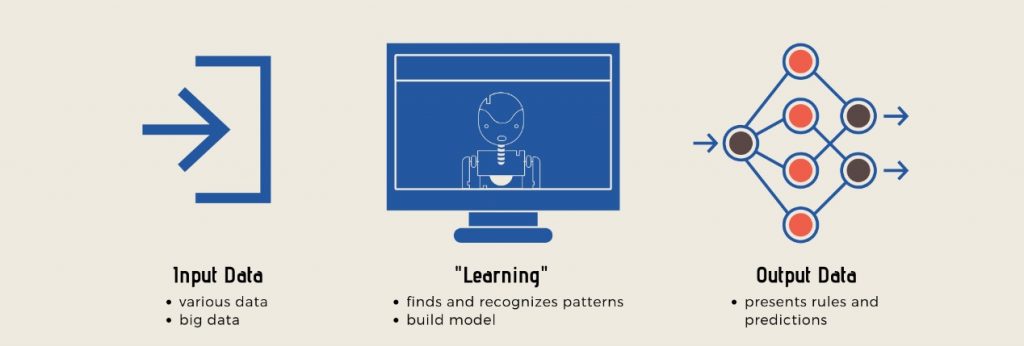
The ability of the system to learn from the data and then apply the insights to new data entering at tremendous speed, without the need for additional resources and human intervention, brings real business value to the development of the organization and the achievement of competitive advantage.
While Artificial Intelligence presents a rather ambitious goal – building a robot that awaits human behavior in all its aspects, and Machine Learning is one of the approaches to realization, we have seen in recent years that Machine Learning has taken a turn and an independent way in which organizations set strategic business goals and achieve them.
Google was a pioneer in this field and is the first company to understand the true value and importance of using Machine Learning in business processes, and since then has only improved its algorithms to better match the needs of its users/customers.
The question arises, how does the company succeed in the difficult task of focusing on the surfing patterns of its billions of surfers? The answer is quite simple – the realization is very complex – by analyzing data accumulated by introducing Machine Learning into its business model.
What is Deep Learning – DL?
Deep Learning is the most talked-about approach recently, in fact, it is a sub-domain or one of the realizations of Machine Learning.
Deep Learning runs an algorithm in a hierarchy of layers, where the output of decisions from one layer enters the next layer.
The guiding principle here is the term “paper networks” based on an understanding of the biological functioning of the human brain – interactions between the neurons in our brain.
However, as opposed to the human brain where each neuron can connect to any other neuron within a certain physical distance, Deep Learning paper networks have separate layers, multiple connections, and different directions, all within a world of multiple data.
The term “deep” describes several layers within the grid so that each layer is more and deeper than the previous one. This model is particularly effective in image processing, text processing, or voice processing.
Uses of Deep Learning in Realtime
Automated Driving: Vehicle researchers use Deep Learning to automatically detect objects such as stop signs and traffic lights. In addition, Deep Learning is used to identify pedestrians, which helps in reducing accidents.
Aerospace and Defense: Deep Learning is used to identify objects from satellites located in areas of interest to identify safe or unsafe areas for soldiers or aircraft.
Medical Research: Cancer researchers use Deep Learning to automatically detect cancer cells. Teams at UCLA have built an advanced microscope that provides a multidimensional dataset used to train deep learning applications to accurately identify cancer cells.
Industrial Automation: The Deep Learning model helps to improve the safety of employees around heavy machinery by automatically identifying a poor area around the machines.
Electronics: The Deep Learning model is used to interpret hearing and automatic speech. For example, different home appliances respond to the voice and know user preferences triggered by Deep Learning applications.
Related Data Science Articles
- How does Machine Learning help the customer behavior detection revolution?
- Machine Learning vs. Deep Learning
- What is Amazon Machine Learning?
- What is Caffe – The Deep Learning Framework
- Guide To Understand The Machine Learning, Deep Learning, AI